Prediction of radiotherapy side effects using explainable AI
In the upcoming years, the PRE-ACT consortium is going to predict the risk of radiotherapy side effects, including arm lymphedema in breast cancer patients by using explainable Artificial Intelligence (AI).
Radiotherapy is a widely used cancer treatment, however some patients suffer side effects. In breast cancer, side effects can include breast atrophy, arm lymphedema, and heart damage. Some factors that increase risk for side effects are known, but current approaches do not use all available complex imaging and genomics data. The time is now ripe to leverage the huge potential of AI towards prediction of side effects. This project will use rich datasets from three patient cohorts to design and implement an AI tool that predicts the risk of side effects, arm lymphedema in breast cancer patients and provides an easily understood explanation to support shared decision-making between the patient and physician.
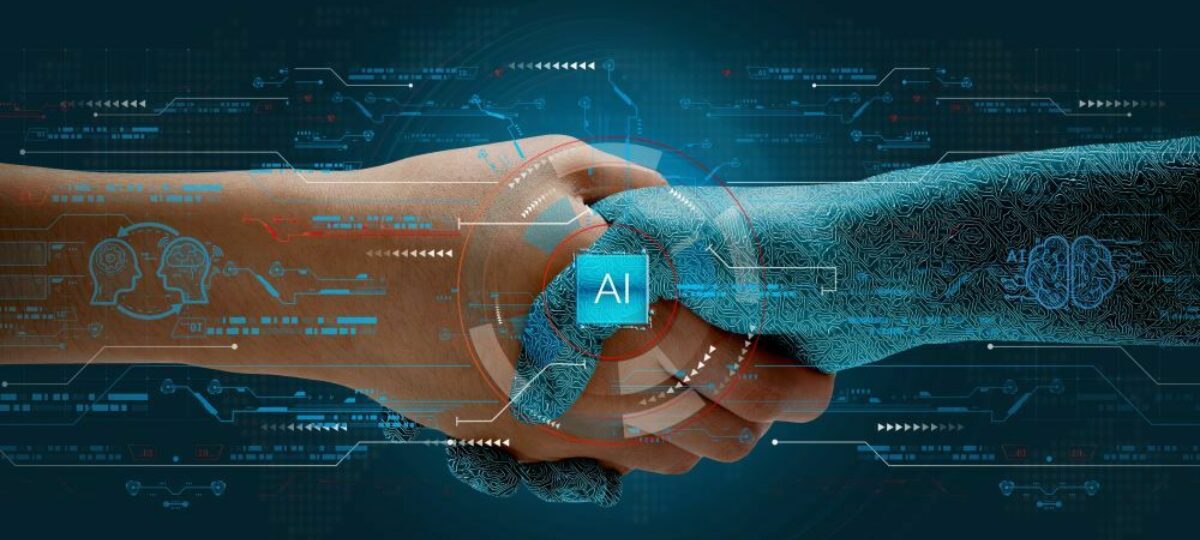
Objectives: breast cancer treatment & arm lymphedema
- Enrich datasets from existing cohorts with additional data that will be collected on patients (e.g. genetic markers in CANTO, cardiac toxicity in REQUITE) to enable the design of integrated AI models with a richer set of features.
- Implement Findability-Accessibility-Interoperability-Reusability (FAIR)-compliant distributed repositories which are needed to perform external validation of the prediction models.
- Create novel local/global “explainability-by-design” methods for deep neural networks and assess the quality of the developed techniques by execution time, clarity of explanations and fidelity.
- Perform federated external validation of the AI-based risk prediction models.
- Adopt a participatory co-creation and co-design process with involved stakeholders (patients, physicians, radiation oncologists) so as to conceive a reference framework of appropriate measures for trustworthy AI in addition to usability, usefulness, acceptability, reliability.
- Evaluate the impact of contouring variability on toxicity prediction within the training cohorts and develop strategies to reduce this in the study cohort.
- Develop and validate accurate AI-based personalised predictive models for key side effects of breast cancer RT including acute skin reactions, late breast and arm toxicity, and cardiac damage. These will improve on existing (mostly empirical and low-dimensional) predictive approaches by including complex imaging and genomic data.
- After training our AI models on a central repository, we will provide proof-of-concept validation for distributed Machine Learning (ML), for cases where data is not transferable; we will take inspiration from Federated Learning and Split Learning which will cope with unique challenges such as feature diversity, population heterogeneity and different class representation in the datasets.
- Make the AI models amenable to generalization for predicting side effects in other cancer types by using Transfer Learning techniques.
- Assess the impact of communicating our AI-based toxicity risk prediction on choice of RT technique and regimen, and ultimately incidence of late toxicity and patient-reported outcomes.
- Include in the predictive models the patient lifestyle and behavioural factors e.g. smoking, alcohol consumption, together with socioeconomic data, where available. Identify and prevent group-specific biases that might constitute potential fairness issues.
- Identify and remove manual biases caused by current, semi-automated methods of CT image contouring analysis by reanalysis using TheraPanacea’s ART-Plan AI-based platform to ensure standardisation across data.
- Design and develop an engaging web/mobile app as part of a trustful information and communication package about AI-based treatment options. The package will support physicians and patients to understand AI model results and take their decisions to modify RT and/or change patient lifestyles to better manage and mitigate side effects.
- Demonstrate the cost-effectiveness of the AI-assisted approach compared with current clinical practice in terms of cost per quality-adjusted life-year (QALY) gained by also considering the utility beyond health outcomes.
Structure (WPs)
- WP1 Management and coordinaton (AUEB-RC)
- WP2 Data management and research infrastructure (MAASTRO)
- WP3 Explainable AI prediction models and AI platform Development (HES-SO)
- WP4 Clinical trial design and implementation for validation of the AI solution (UNICANCER)
- WP5 Stakeholder co-design and communication package (CNR)
- WP6 Fair, Transparent and Ethical AI (CENTAI)
- WP7 Health Economics (UM)
- WP8 Communication, Dissemination and Exploitation (MAASTRO)
Read more about Structure (WPs)
Consortium
The consortium consists of a highly interdisciplinary team with complementary expertise and prior collaboration record (6 world-leading research partners, 2 SMEs, 1 radiotherapy institute and 1 hospital network), from six countries: Greece, France, UK, Italy, Netherlands, and Switzerland. The consortium covers the whole value chain from AI development until exploitation in the market.
Read more about the Consortium
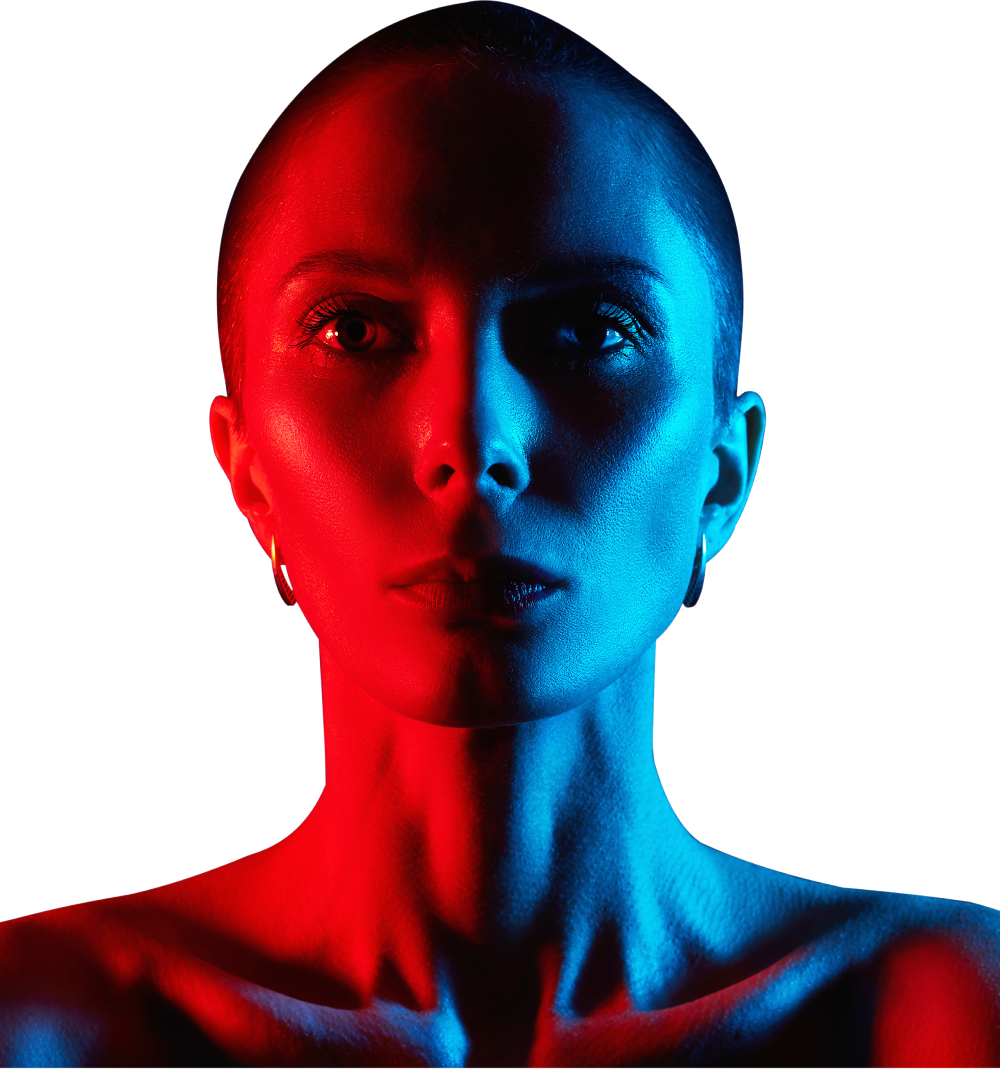
Enabling clinical trials
Explainable AI with improved predictive power for radiotherapy toxicity will enable clinical trials in which the AI is used to stratify patients for treatment modification. It will also lead to a widespread radical change of clinical practice on how crucial decisions are taken, especially in the first stages of cancer treatment. Future examples will include: (i) giving suitable patients hypofractionated radiotherapy with a reduced number of larger doses, which could lead to reduced side-effects and is less time-consuming for patients; (ii) treating early stage breast tumours with partial breast irradiation in patients at high risk for side effects; (iii) advising selected patients whether to have a mastectomy (thus avoiding radiotherapy) or a reduction mammoplasty prior to radiotherapy; (iv) deciding whether to use conventional photon radiotherapy or more expensive proton therapy.
Click here for more information on the clinical trial